Chithra Srinivasan
Einstein Analytics & Machine Learning Model
-
Led the application team in the implementation of Data Science, Machine Learning Model using Sales cloud computing for Generation of Einstein Analytics Predictions and Insights
-
Leveraged the Tableau CRM Plus capabilities in building the Recipes,Story,Generation of Insights and Predictions and successful deployment techniques.
-
Collaboration with Technical & Business Architecture teams, Environment and Release Team for sprint planning and User Story Creation
-
Deployment of Classification Analytical Model in Production and Maintenance through Automatic Refresh and Anchored the Build of Lightning Flows to update Scores and other results in Custom object
ML Classification & Numerical Prediction Python Model
-
Demonstrated ability in Data Modeling and Guided the Data Engineering team in Data Extraction process- Reviewed and resolved SQL stored procedures issues.
-
Collaborated with cross-functional teams to define project requirements and translate business problems into data science solutions
-
Conducted exploratory data analysis and applied statistical techniques to identify patterns and trends in large datasets
-
Removed Data Anomalies and applied suitable Machine Learning and feature selection techniques for the model.
-
Presented findings and recommendations to stakeholders through PowerBi-Visualization widgets along with predictions for unknown data
Other Projects
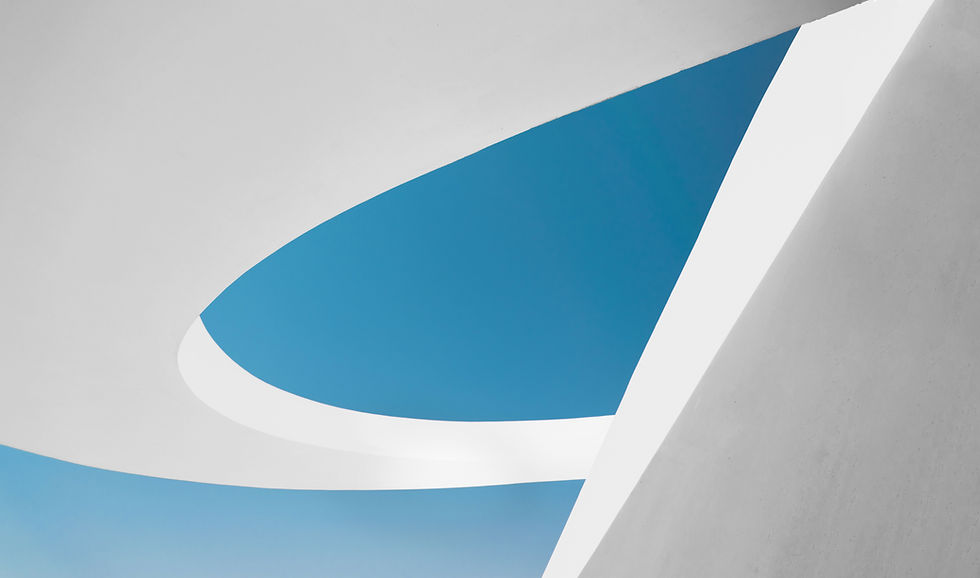
1
Salesforce Data Modeling and Dashboard
-
Recommendation and Decision Making Tool for Deal Pricing Team
-
Data Modeling and Data Preparation
-
Estimating Pricing Ranges across the segments
-
Historical Pricing Insights through Salesforce Dashboard Capacity
2
PowerBI Dashboard
-
Optimization engine for devising strategy to revise the targets
-
Insights on Historical Deal Targets and Recommendations for Revision of Targets
-
Comparison of Actual vs Target Metrics across various segmentations
3
Data Analytics and Data Governance
-
Generation of Risk Weighted Average Parameters and Basel III Implementation
-
Guidance on Data Modeling Requirements to the data ingestion, acquisition and engineering teams
-
Data descriptive statistics for credit risk portfolios, Trend Charts and Visualizations